Nine Opportunities and Risks of Algorithmic Decision-Making
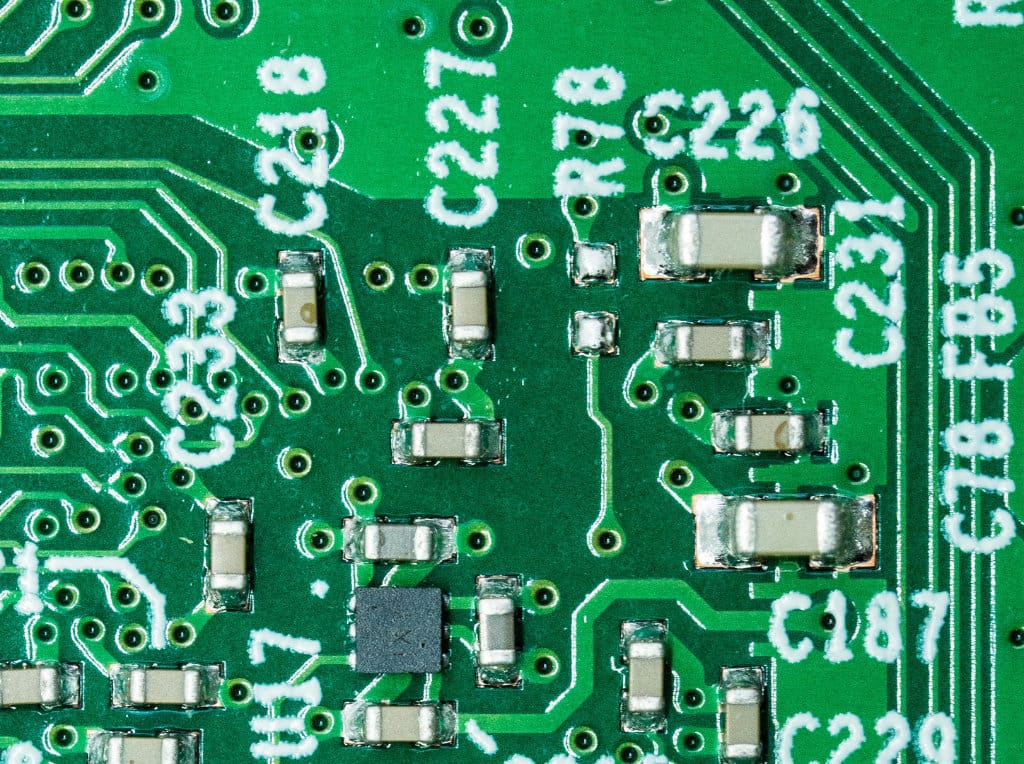
Processes of algorithmic decision-making (ADM) now evaluate people in many areas of life. ADM processes have been used for years to categorize people, without any real discussion of whether those processes are fair or how they can be explained, verified or corrected. One potential reason for this is that the systems have little to do with artificial intelligence (AI) as it appears in science fiction. People often associate AI with qualities exhibited by fictional characters like HAL 9000 or Wintermute: intentionality and consciousness. Yet, until now, powerful AIs of this sort have only been found in literary works and films, and have nothing to do with the systems presented in this collection of case studies. The latter, however, already play a significant role in deciding legal matters, approving loans, admitting students to university, determining where and when police officers are on duty, calculating insurance rates and assisting customers who call service centers. All are programs which are specially designed to address specific problems and which impact the lives of many people. It’s not about the future according to science fiction, it’s about everyday reality today.
To take advantage of the opportunities ADM offers in the area of participation, one overall goal must be set when ADM processes are planned, designed and implemented: ensuring that participation actually increases. If this is not the case, the use of these tools could in fact lead to greater social inequality. Here is a summary of opportunities and risks found in case studies:
1. Normative principles
Opportunity: When an ADM process is designed, normative decisions (e.g. about fairness criteria) must be made before the process is used. This offers an opportunity to discuss ethics issues thoroughly and publically at the very start and to document decisions.
Risk: ADM processes can contain hidden normative decisions. If discussion is only possible once the design phase is complete, any normative principles are more likely to be accepted as unalterable
2. Data
Opportunity: Software can analyze a much greater volume of data than humans can, thereby identifying patterns and answering certain questions faster, more precisely and less expensively..
Risk: The data used for an ADM process can contain distortions that are seemingly objectified by the process itself. If the causalities behind the correlations are not verified, there is a significant danger that unintentional, systematic discrimination will become an accepted part of the process.
3. Consistency of application
Opportunity: Algorithm-based predictions apply the predetermined decision-making logic to each individual case. In contrast to human decision makers, software does not have good and bad days and does not in some cases arbitrarily use new, sometimes inappropriate criteria.
Risk: In exceptional cases, there is usually no possibility for assessing unexpected relevant events and reacting accordingly. ADM systems unfailingly make use of any incorrect training data and faulty decision-making logic.
4. Scalability
Opportunity: Software can be applied to an area of application that is potentially many times larger than what a human decision maker can respond to, since the decision-making logic used in a system can be applied at very low cost to a virtually limitless number of cases.
Risk: ADM processes are easily scalable, which can lead to a decrease in the range of such processes that are or can be used, and to machine-based decisions being made much more often and in many more instances that might be desirable from a societal point of view
5. Verifiability
Opportunity: Data-driven and digital systems can be structured in a way that makes them clear and comprehensible, allows them to be explained and independently verified, and provides the possibility of forensic data analysis.
Risk: Because of process design and operational application, independent evaluations and explanations of decisions are often only possible, comprehensible or institutionalized to a limited degree.
6. Adaptability
Opportunity: ADM processes can be adapted to new conditions by using either new training data or self-learning systems
Risk: The symmetry of the adaptability in all directions depends on how the process is designed. One-sided adaptation is also possible.
7. Efficiency
Opportunity: Having machines evaluate large amounts of data is usually cheaper than having human analysts evaluate the same amount.
Risk: Efficiency gains achieved through ADM processes can hide the fact that the absolute level of available resources is too low or inadequate.
8. Personalization
Opportunity: ADM processes can democratize access to personalized products and services that for cost-related reasons were previously only available to a limited number of people. For example, before the Internet, numerous research assistants and librarians were required to provide the breadth and depth of information that results from a single search-engine query.
Risk: When ADM processes are the main tools used for the mass market, only a privileged few have the opportunity to be evaluated by human decision makers, something that can be advantageous in non-standard situations when candidates are being preselected or credit scores awarded.
9. Human perception of machine-based decisions
Opportunity: ADM processes can be very consistent in making statistical predictions. In some cases, such predictions are more reliable than those made by human experts. This means software can serve as a supplementary tool which frees up time for more important activities.
Risk: People can view software-generated predictions as more reliable, objective and meaningful than other information. In some cases this can prevent people from questioning recommendations and predictions or can result in their reacting to them only in the recommended manner.
An important – even definitive – quality factor must be stressed once again: An analysis of the opportunities, risks and societal consequences was only possible because independent third parties were able to verify the benefits of machine-based decisions. Institutions such as the investigative newsroom ProPublica, the US Government Accountability Office and the student-rights organization Droits des lycéens spent the time and financial resources needed to collect and evaluate data and to consider the relevant legal issues, allowing each of the algorithms to be explained and made transparent. Public debate on the impact of certain ADM processes thus depends completely on institutions of this sort – a situation that must change. It must be possible to verify and understand algorithmic decisions if an effective discussion is to take place, one which ensures that ADM processes actually increase participation and that machine-based decisions truly benefit people. ADM processes will only contribute to the common good if they are discussed, criticized and corrected. We are still in a position to determine how we as a society want to make use of algorithms. We should not only consider how they are applied, but, in some cases, whether they should be used at all. For example, in those situations where society has chosen to promote solidarity and share risks, ADM processes cannot be permitted to individualize those risks. The guiding principle cannot be what is technically feasible, but what makes sense from a societal perspective – so that machine-based decisions truly do benefit people.
This is an excerpt from the working paper “Wenn Maschinen Menschen bewerten — Internationale Fallbeispiele für Prozesse algorithmischer Entscheidungsfindung”, written by Konrad Lischka and Anita Klingel, published by the Bertelsmann Stiftung under CC BY-SA 3.0 DE.
This publication documents the preliminary results of our investigation of the topic. We are publishing it as a working paper to contribute to this rapidly developing field in a way that others can build on.